Chapter 6 Guyafor
In this chapter, I repeated the model fit for the whole Guyafor network.
6.1 Data
I used only recruited trees in the censuses with at least 4 measurements of diameter at breast height (DBH, cm). I used only species with at least 4 trees following previous requirements (Tab. 6.1 & Fig. 6.1).
N | Median | Minimum | Maximum | |
---|---|---|---|---|
families | 49 | |||
genera | 155 | |||
species | 297 | |||
individuals | 21 769 | |||
observations | 267 530 | |||
census | 12 | 5 | 30 | |
year0 | 1 998 | 1 986 | 2 011 | |
yearmax | 2 021 | 1 997 | 2 021 | |
dbh0 | 11 | 5 | 15 | |
dbhmax | 14 | 6 | 83 |
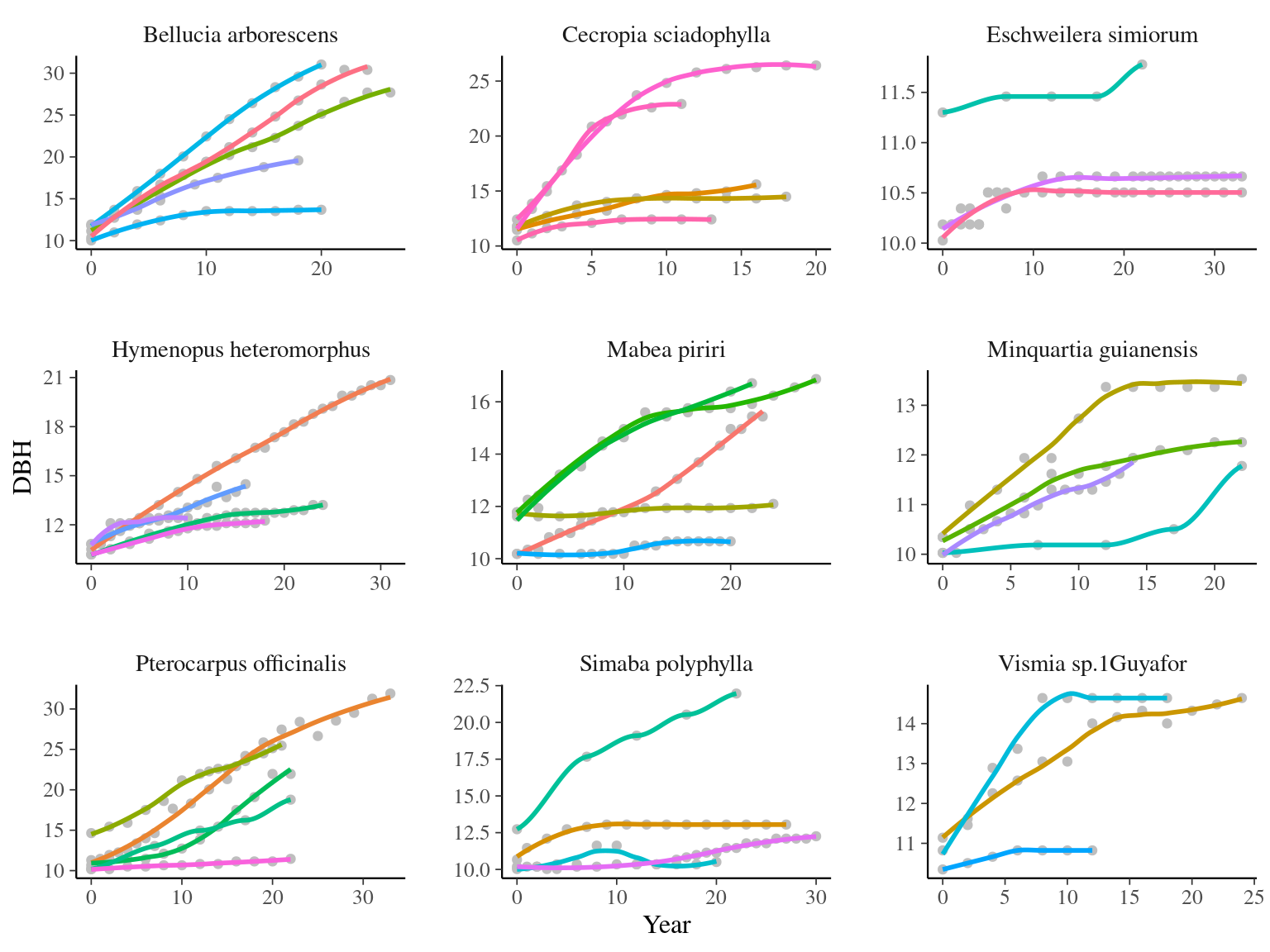
Figure 6.1: Tree diameter trajectories in reduced data. Color represent individuals.
6.2 Model
I used a Gompertz model (Herault2011?), were the diameter of individual \(i\) at year \(t\) is the sum of annual growth from \(t0\) to \(t\):
\[ DBH_{t,i,s} \sim \mathcal N (10 + Gmax_i \times \sum _{y=1|DBH_{t=0}} ^{y=t} exp(-\frac12.[\frac{log(\frac{DBH_{t,i}}{100.Dopt_i})}{Ks_i}]^2)), \sigma) \\| Dopt_i \sim \mathcal N(Dopt_s,\sigma_D), Ks_i \sim \mathcal N(Ks_s,\sigma_K) \]
The annual growth rate for individual \(i\) at year \(y\) with a diameter of \(DBH_{y,i}\) is defined following a Gompertz model (Gompertz 1825) already identified as the best model for growth-trajectories in Paracou (Herault2011?), where \(Gmax_i\) is the fixed maximum growth potential of every individual, \(Dopt_i\) is the optimal diameter at which the individual reaches its maximum growth potential, and \(Ks_i\) is the kurtosis defining the width of the bell-shaped growth-trajectory (see figure 1 in Herault2011?). \(Dopt_i\) and \(Ks_i\) are random effects centered on species parameters \(Dopt_s\) and \(Ks_s\) with associated variances \(\sigma_D\) and \(\sigma_K\).
fit <- read_cmdstan_csv("save/growthguyafor/growth-202210280057-1-361cf6.csv")
draws <- drop(fit$post_warmup_draws) %>%
as_data_frame() %>%
mutate(iteration = 1:n()) %>%
gather(parameter, value, -iteration)
vroom::vroom_write(draws, file = 'save/growthguyafor/chain1.tsv')
rm(fit, draws)
gc()
fit <- read_cmdstan_csv("save/growthguyafor/growth-202210280057-2-361cf6.csv")
draws <- drop(fit$post_warmup_draws) %>%
as_data_frame() %>%
mutate(iteration = 1:n()) %>%
gather(parameter, value, -iteration)
vroom::vroom_write(draws, file = 'save/growthguyafor/chain2.tsv')
rm(fit, draws)
gc()
fit <- read_cmdstan_csv("save/growthguyafor/growth-202210280057-3-361cf6.csv")
draws <- drop(fit$post_warmup_draws) %>%
as_data_frame() %>%
mutate(iteration = 1:n()) %>%
gather(parameter, value, -iteration)
vroom::vroom_write(draws, file = 'save/growthguyafor/chain3.tsv')
rm(fit, draws)
gc()
fit <- read_cmdstan_csv("save/growthguyafor/growth-202210280057-4-361cf6.csv")
draws <- drop(fit$post_warmup_draws) %>%
as_data_frame() %>%
mutate(iteration = 1:n()) %>%
gather(parameter, value, -iteration)
vroom::vroom_write(draws, file = 'save/growthguyafor/chain4.tsv')
rm(fit, draws)
gc()
grep gmax chain1.tsv > gmax1.tsv
grep gmax chain2.tsv > gmax2.tsv
grep gmax chain3.tsv > gmax3.tsv
grep gmax chain4.tsv > gmax4.tsv
bind_rows(
vroom::vroom('save/growthguyafor/gmax1.tsv', col_names = c("iteration", "parameter", "value")) %>%
mutate(chain = 1),
vroom::vroom('save/growthguyafor/gmax2.tsv', col_names = c("iteration", "parameter", "value")) %>%
mutate(chain = 2),
vroom::vroom('save/growthguyafor/gmax3.tsv', col_names = c("iteration", "parameter", "value")) %>%
mutate(chain = 3),
vroom::vroom('save/growthguyafor/gmax4.tsv', col_names = c("iteration", "parameter", "value")) %>%
mutate(chain = 4)
) %>%
separate(parameter, c("gmax", "ind")) %>%
vroom::vroom_write(file = 'save/growthguyafor/gmax.tsv')
6.3 Fit
Unfortunately, gmax upper limit was set to 5 instead of 10 (overconstrained). But the model with more stringent data had a better behavior with gmax limited to 5.
6.4 Evolutionary analyses
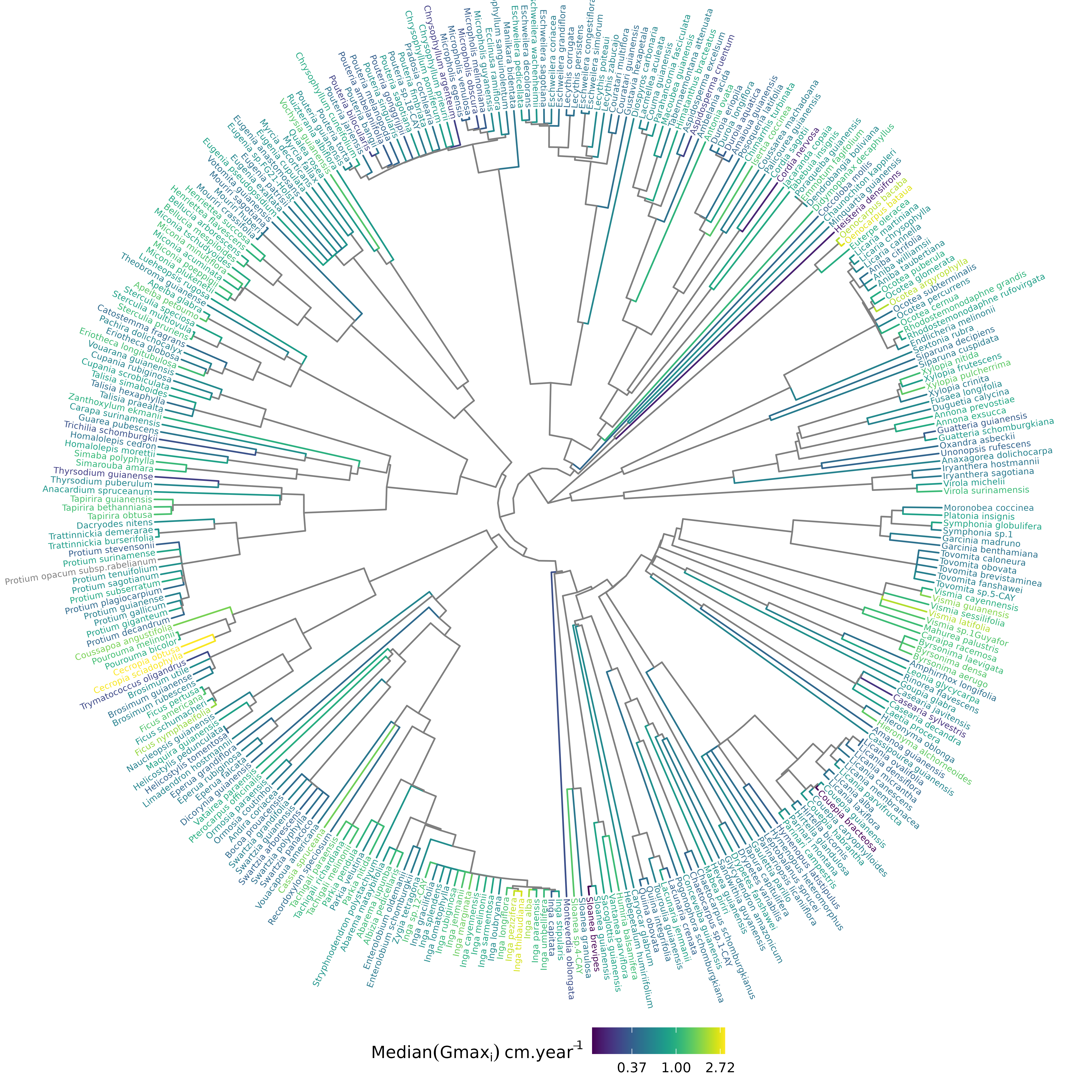
Figure 6.2: Distribution of species growth potential (Gmax, cm/yr) in the phylogeny.
6.5 Discussion
- Within-chain parallelization available with gompertzsumpar model.
- Next steps:
- Stop?
- Use?
- Run again?
- Cluster?
- Who?
- When?
- What for? Data paper?